The Nevada DRIVE Program is a two-year graduate research assistantship (GRA) program made possible through the combined efforts of President Brian Sandoval, Vice President for Research and Innovation, Mridul Gautam, and Executive Vice President & Provost, Jeff Thompson.
Applications for this program are now closed.
“The privileges the DRIVE scholarship has given me are enormous. I am particularly excited about how DRIVE manages the students and provides all kinds of resources to scholars for them to be successful. I have met an exciting group of researchers through this program who have widened my interest in research. I am hoping to contribute to the world of research and provide clean and healthy drinking water worldwide.” –Emmanuel Cobbinah, Hydrogeology doctoral student
Purpose
To provide one- or two-year Graduate Research Assistantships to promote the recruitment/retention of doctoral students enrolled at the University of Nevada, Reno.
Program description
The Nevada DRIVE program is designed to promote Doctoral Research in Innovation, Vision and Excellence at the University of Nevada, Reno. All University doctoral programs are eligible to request funding for one or more full- or half- time GRA positions for the 2023-2024 and 2024-2025 academic years. For each year, we anticipate that funding will be available to support approximately 20 full-time (20-hour/week) or 40 half-time (10-hour/week) research assistantships with a stipend of $25,000, or $12,500, respectively. Funding includes non-resident tuition waivers and the standard grant-in-aid to cover course registration fees and student health insurance.
Please refer to the University’s Graduate Assistant Handbook for information on policies, benefits, and definitions regarding graduate assistants. For the purposes of this program, a graduate research assistant is broadly defined below.
Graduate Research Assistant (GRA): GRAs are graduate assistants who work in research-related positions existing primarily in academic departments, research centers and institutes. GRAs gain experience of working alongside faculty members on cutting-edge research.
GRA responsibilities vary greatly and may include, but are not limited to:
- Conducting research or performing creative activities towards the completion of their doctoral degree
- Collecting, coding, and/or analyzing data
- Conducting literature reviews or library research
- Preparing materials for submission to funding agencies and foundations
- Writing reports
- Preparing materials for IRB review
Program requirements
The following program requirements must be met by the graduate program, faculty member, and/or graduate student during the funding year(s).
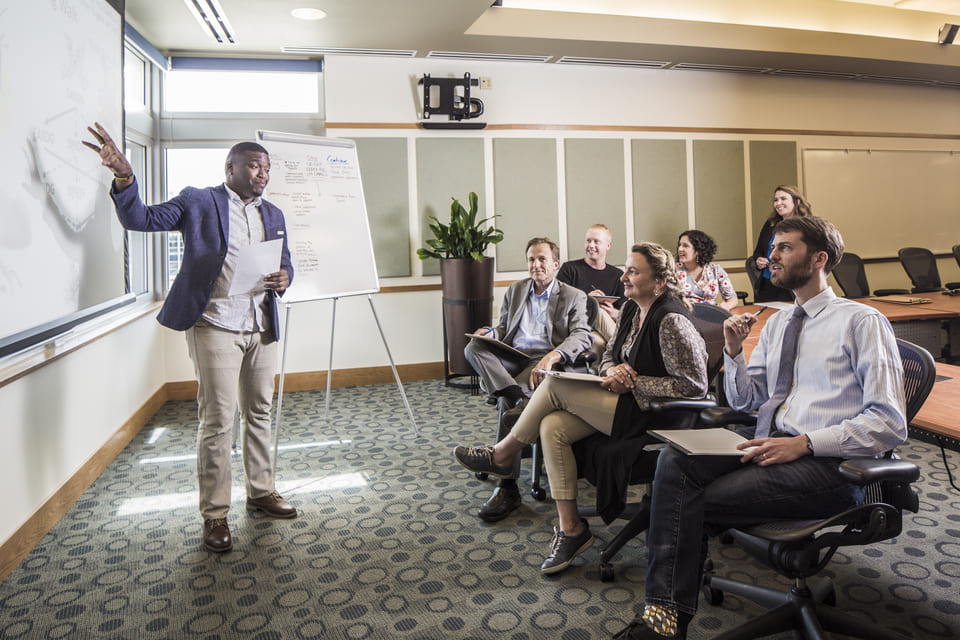
Incoming Nevada DRIVE Scholars
- The incoming Nevada DRIVE scholar must submit an individual development plan (IDP) by the end of Fall 2023 (for Fall 2023 admits) or the end of Fall 2024 (for Fall 2024 admits).
- If appropriate, the incoming Nevada DRIVE scholar must apply to the NSF GAIN Scholars Program (refer to NSF GAIN Scholars webpage for eligibility)
All Nevada DRIVE Scholars
- The Nevada DRIVE Scholar must attend a mandatory orientation session at the beginning of Fall 2023 or Fall 2024 semesters, as appropriate.
- The graduate program must assign a peer mentor to the Nevada DRIVE Scholar. The peer mentor should be an established doctoral student in the Nevada DRIVE Scholar’s graduate program.
- The Nevada DRIVE Scholar must attend structured seminars and professional development modules to investigate and prepare for careers.
- The Nevada DRIVE Scholar must receive additional mentorship with a University faculty member other than their doctoral advisor.
- The Nevada DRIVE Scholar must participate in at least one of the following programs: GradFIT, Gradventure, GSA Poster Symposium, and/or 3MT.
All Nevada DRIVE Advisors
- The faculty advisor must submit an annual evaluation of progress for the Nevada DRIVE Scholar to the Graduate School by May 2024 and/or May 2025.
- The Nevada DRIVE Scholar’s doctoral advisor must complete or have completed the Graduate School’s Mentoring Mentor’s Workshop.